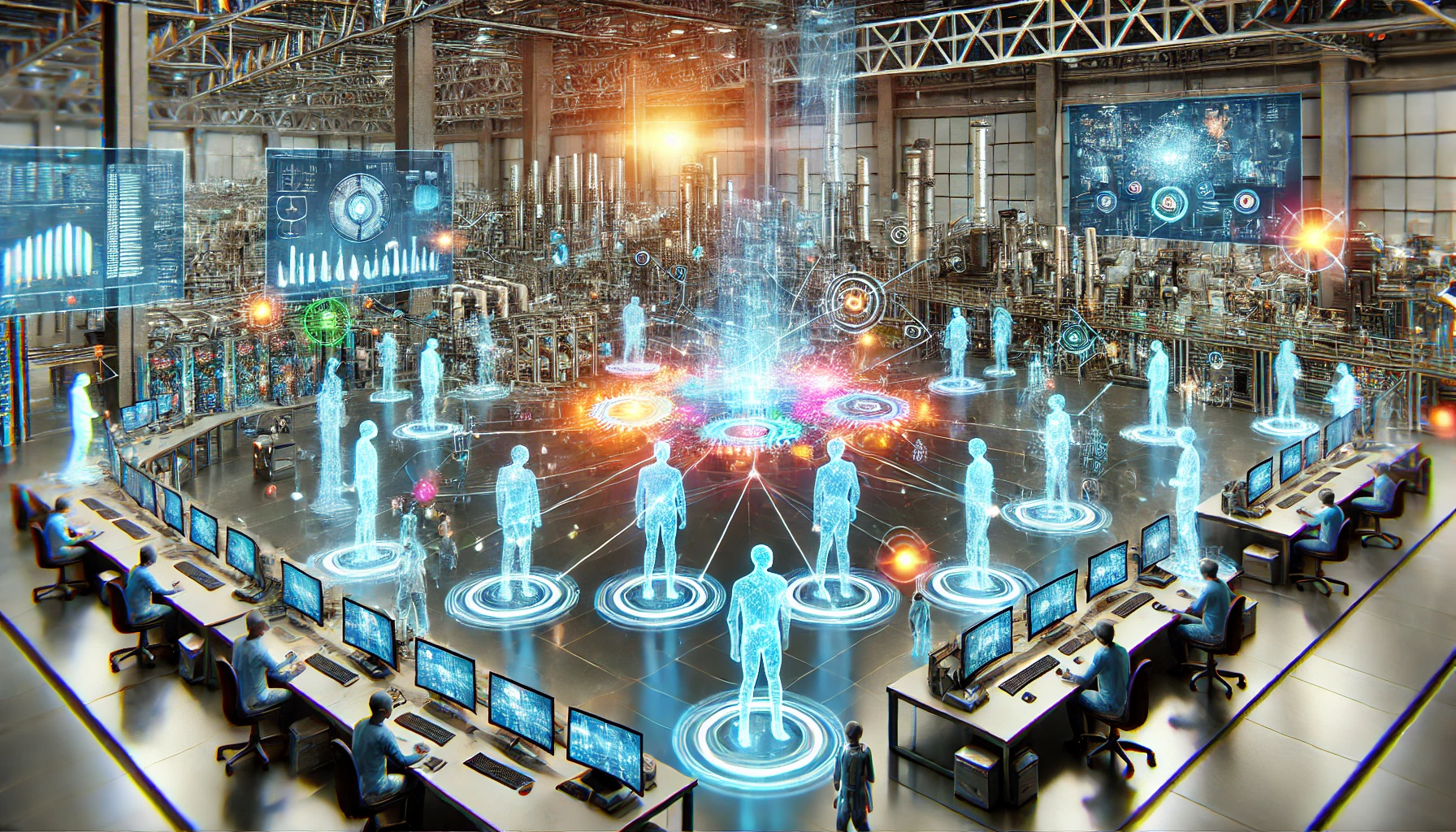
XMPro Presents:
The Ultimate Guide to Multi-Agent Generative Systems
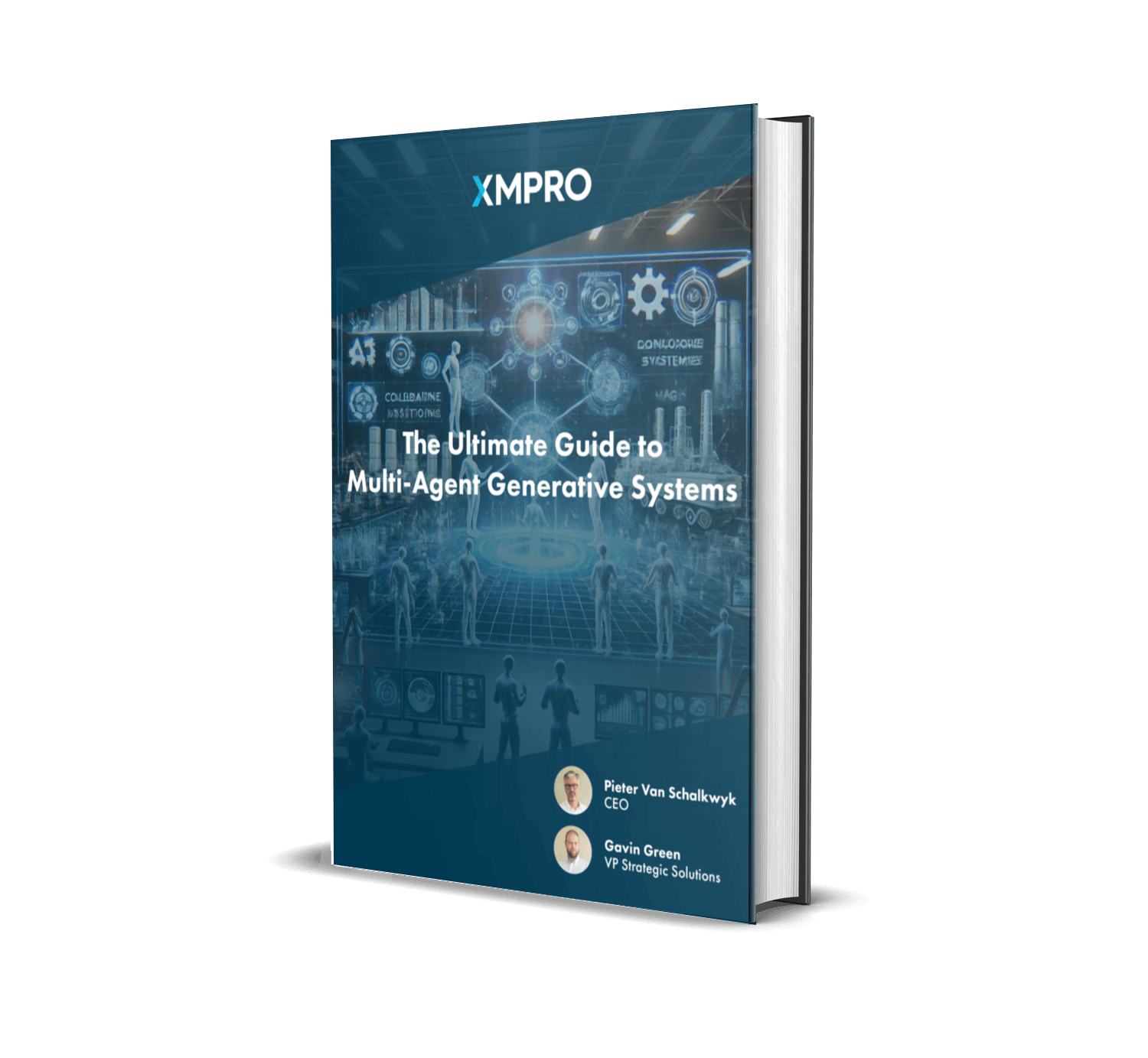
Table of Contents
1.Introduction
The Rise of Generative AI and Intelligent Agents
Generative AI and intelligent agents have recently emerged as transformative forces in the field of artificial intelligence. These technologies are not only changing how we interact with machines but also revolutionizing complex processes across multiple industries. Generative AI is renowned for its ability to produce new content, ideas, and solutions by drawing upon vast amounts of data, offering an unparalleled level of creativity. This has gained widespread attention in areas like text generation, content creation, and even creative coding. On the other hand, intelligent agents function autonomously, making real-time decisions and taking actions based on their observations, goals, and internal models of the world around them. These agents are increasingly being deployed to optimize operations in dynamic environments such as manufacturing plants, energy grids, logistics, and even customer service.
💡 An XMPro AI agent is an industrial-grade cognitive entity that combines memory cycles, specialized knowledge, and advanced reasoning capabilities to continuously observe, reflect, plan, and act within complex industrial environments.
The convergence of these two technologies—generative AI and intelligent agents—has given birth to Multi-Agent Generative Systems – MAGS, a new and powerful class of AI systems. MAGS harness the creative potential of generative models while enabling intelligent agents to collaborate, adapt, and solve complex problems in real time. This new synergy elevates AI systems beyond traditional automation, pushing them into the realm of autonomous problem-solving, creative thinking, and adaptive decision-making.
The development of MAGS marks a significant shift in the way organizations approach challenges in rapidly evolving environments. By integrating Large Language Models (LLMs) and other advanced generative models with agents capable of learning and interacting independently, MAGS enable organizations to address problems that are not only multifaceted but also constantly changing. This guide explores the foundational elements of MAGS, their architecture, and why they matter for industries that need to navigate complexity, unpredictability, and scale.
What Are Multi-Agent Generative Systems?
At the heart of Multi-Agent Generative Systems – MAGS is the concept of collaboration between multiple autonomous agents. Each agent operates within its environment, processing inputs, learning from past experiences, and making decisions. However, MAGS are not limited to basic decision-making; they go beyond by incorporating generative AI models. This integration allows agents to generate entirely new ideas, strategies, or content based on their objectives and the context of their environment.
Imagine a factory floor where various AI agents are assigned specific roles: one monitors machinery performance, another oversees supply chain logistics, and a third forecasts product demand. In a traditional system, these agents would be bound by pre-programmed rules and behaviors. In a MAGS, however, these agents can engage in creative problem-solving by generating new solutions to unforeseen challenges. For example, if a machine unexpectedly breaks down, a MAGS agent could analyze the situation, propose a new maintenance schedule, and even suggest alternative production routes—all while coordinating with other agents in real time.
💡 MAGS is an industrial-grade framework where specialized AI agents work collaboratively under clear protocols to optimize complex processes, sharing knowledge and coordinating actions in real-time.
XMPro MAGS: A Unique Approach
XMPro MAGS stands out in this evolving landscape by offering a highly sophisticated approach tailored to large-scale industrial operations and enterprise environments. The XMPro MAGS platform introduces a range of advanced features designed to ensure that agents operate not only autonomously but also collaboratively, continuously learning from their environment. This dynamic system is built to optimize complex workflows, improve decision-making, and streamline operations in mission-critical settings.
What is XMPro MAGS? XMPro MAGS are dynamic teams of virtual workers powered by advanced artificial intelligence. These self-organizing teams work independently and collaboratively to optimize operational outcomes and achieve specified goals.
Some of the key features of XMPro MAGS include the following:

Autonomous Decision-Making:
Each agent in XMPro MAGS functions independently, analyzing data, making decisions, and executing tasks without human intervention. This autonomy allows agents to react swiftly to changes in their environment while maintaining alignment with overall operational goals.
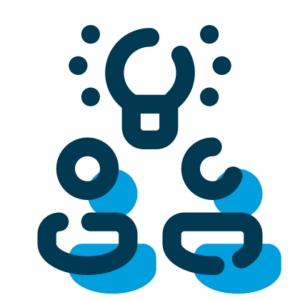
Collaborative Problem-Solving:
While each agent has a defined role, they are also capable of working together to tackle more complex challenges. The system enables multi-agent collaboration, where agents share insights, exchange information, and coordinate actions to optimize system-wide performance.
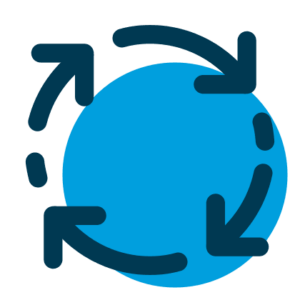
Advanced Memory Cycles:
XMPro MAGS agents are equipped with memory cycles that enable them to store and retrieve information, recall past experiences, and learn from them. This means that each agent has access to a historical context that informs its future decisions, making it more capable of long-term planning and adaptation.
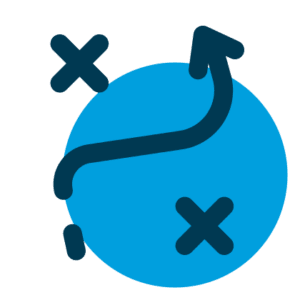
Flexible Planning Strategies:
The platform supports multiple planning approaches, including the use of Planning Domain Definition Language (PDDL), allowing agents to tackle a wide variety of complex scenarios. This adaptability is essential for environments where conditions can change quickly and unexpectedly.
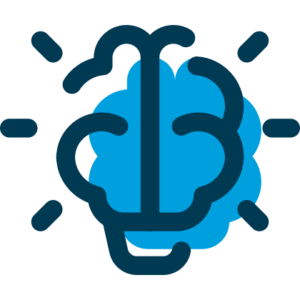
Generative Creativity:
What truly sets XMPro MAGS apart is its ability to leverage generative AI. By utilizing LLMs and other generative models, agents in XMPro MAGS can generate new ideas, solutions, and strategies in real-time, far beyond the confines of static rule-based systems.
XMPro MAGS is designed to meet the needs of industries with complex operations, where the ability to rapidly adapt and optimize workflows is crucial for maintaining operational efficiency and competitiveness. By combining autonomous decision-making with creative problem-solving, XMPro MAGS offers organizations a new level of operational intelligence.
Why MAGS Matter
The emergence of Multi-Agent Generative Systems MAGS represents a pivotal advancement in the field of artificial intelligence. MAGS address many of the limitations of traditional AI systems, particularly in environments where complexity, variability, and the need for real-time decision-making are paramount. In industries such as manufacturing, supply chain management, and energy production, MAGS provide a level of adaptability, creativity, and scalability that was previously unattainable.
Enhanced Creativity and Problem-Solving:
Traditional systems often rely on predefined rules to guide their actions, making them ill-suited for handling novel or unexpected situations. MAGS, on the other hand, excel at creative problem-solving by leveraging generative models. By utilizing tools like LLMs, agents can dynamically generate new strategies and solutions that respond to the specific needs of the situation. This allows them to address challenges in ways that static systems simply cannot.
Real-Time Adaptability:
The ability to adapt in real time is one of the most critical advantages of MAGS. In industries where conditions and inputs change rapidly—such as logistics or energy management—MAGS agents continuously monitor their environment and adjust their behavior accordingly. The integration of generative models enables them to react not only by following predefined protocols but by generating new responses as situations evolve.
Scalability and Collaboration:
MAGS are designed to scale across large, complex systems. By deploying multiple agents with specialized roles, the system can manage vast operations, from global supply chains to intricate industrial processes. Each agent operates autonomously, yet their collaborative nature allows for efficient division of tasks, knowledge sharing, and joint decision-making. This ensures that even in large-scale environments, MAGS maintain optimal performance.
Improved Decision-Making:
MAGS are built upon sophisticated cognitive reasoning models, such as the Observe, Reflect, Plan, Act (ORPA) cycle, which enables agents to make informed decisions. By continuously observing their environment, reflecting on past actions, planning future strategies, and executing tasks, MAGS agents exhibit a level of intelligence that allows for continuous improvement. This process ensures that decision-making is not only informed by historical data but also adaptable to new inputs.
Improved Decision-Making:
MAGS are built upon sophisticated cognitive reasoning models, such as the Observe, Reflect, Plan, Act (ORPA) cycle, which enables agents to make informed decisions. By continuously observing their environment, reflecting on past actions, planning future strategies, and executing tasks, MAGS agents exhibit a level of intelligence that allows for continuous improvement. This process ensures that decision-making is not only informed by historical data but also adaptable to new inputs.
Seamless Integration with Existing Systems:
One of the most practical benefits of MAGS is their ability to integrate seamlessly with existing business and industrial systems. Whether working with IoT devices, real-time data streams, or legacy infrastructure, MAGS can be incorporated into an organization’s current operations without the need for disruptive overhauls. XMPro MAGS, for example, provides the tools necessary to connect with diverse data sources and platforms, enabling businesses to leverage the power of generative AI without replacing their current systems.
Multi-Agent Generative Systems – MAGS are more than just a technological innovation; they represent a new way of thinking about automation, creativity, and problem-solving in dynamic environments. As industries continue to face increasingly complex challenges, the ability to generate novel solutions, collaborate at scale, and adapt in real time is becoming a necessity rather than a luxury. By combining the strengths of generative AI with autonomous, collaborative agents, MAGS offer a flexible, scalable, and highly intelligent solution to the challenges of the modern world.
XMPro MAGS exemplifies these advancements, offering a platform that is not only adaptable and scalable but also deeply integrated with the real-time needs of industries. With its ability to integrate seamlessly into existing operations, XMPro MAGS empowers organizations to make the leap from traditional automation to a future driven by autonomous, creative, and adaptive AI systems.
II. Core Components of MAGS
Agent Architecture
The foundation of any Multi-Agent Generative System lies in its agent architecture. This architecture defines the structure, capabilities, and interactions of individual agents within the system. A well-designed agent architecture is crucial for achieving the desired level of intelligence, autonomy, and collaboration in a MAGS.
Key components of a MAGS agent architecture include the following:
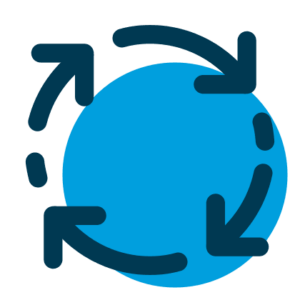
Memory Cycle:
A sophisticated memory management system that allows agents to store and process various types of memories, including observations, reflections, plans, decisions, and actions.
Enables agents to maintain a rich historical context and learn from past experiences.
The MemoryCycle class in XMPro MAGS demonstrates this functionality, showcasing the platform’s ability to handle diverse memory types and their associated metadata.
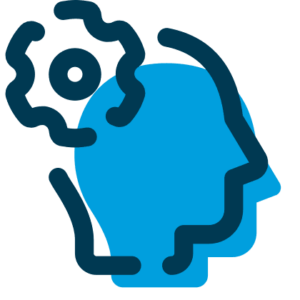
Reflection and Planning:
Agents possess the capability to reflect on their experiences, generate higher-level insights, and formulate plans based on these reflections.
This meta-cognitive ability enables continuous learning and adaptation, crucial for long-term deployment in dynamic environments.
The ReflectAsync and GeneratePlanAsync methods in XMPro MAGS’ MemoryCycle class showcase this functionality.
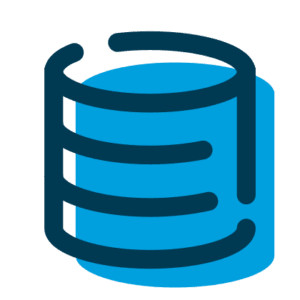
Vector-Based Memory Retrieval:
Integration with vector databases allows for efficient similarity-based retrieval of memories.
This is crucial for agents to quickly access relevant past experiences in large-scale operations, enabling them to make informed decisions based on similar situations encountered previously.
XMPro MAGS integrates with various vector databases, including Milvus, Qdrant, and MongoDB Atlas, demonstrating the platform’s flexibility and scalability in handling large volumes of memory data.
These core components work together to create intelligent, adaptive, and collaborative agents that form the building blocks of a MAGS. The memory cycle provides the foundation for learning and context-aware decision-making, while reflection and planning capabilities enable agents to strategize and optimize their actions. Vector-based memory retrieval ensures efficient access to relevant information, even in large-scale deployments with vast amounts of data.
XMPro MAGS exemplifies these architectural principles, showcasing a sophisticated agent design that is well-suited for complex industrial and enterprise environments. Its comprehensive memory management, multi-tool support, advanced planning capabilities, and robust communication infrastructure make it a compelling choice for organizations looking to leverage the full potential of MAGS.
Multi-Tool Support
In the context of Multi-Agent Generative Systems, multi-tool support refers to the ability of agents to access and utilize a diverse range of tools to enhance their capabilities and perform various tasks. These tools can be internal functions, external APIs, or integrations with other systems, providing agents with the flexibility to address a wide array of challenges and scenarios.
Tool Library
A key component of multi-tool support is the Tool Library, which acts as a centralized repository for managing and organizing the various tools available to agents. The Tool Library allows agents to:
- Access a diverse collection of tools, including those for data analysis, language processing, web searching, and more.
- Select the most appropriate tool for a given task based on its capabilities and requirements.
- Invoke tools with specific parameters and receive results in a standardized format.
XMPro MAGS features a robust Tool Library that provides agents with access to a wide range of tools, including:
- Built-in tools such as SQL query tools, web search tools, sentiment analysis tools, and more.
- Access to existing XMPro DataStreams, providing access to XMPro’s extensive library of 200+ connectors and DataStream tools.
- The ability to integrate with external APIs and services, allowing agents to interact with a wide range of external systems.
This enables agents to perform complex tasks and gather information from various sources, enhancing their problem-solving capabilities.
Dynamic Tool Loading
Dynamic tool loading refers to the ability of the MAGS platform to load and initialize tools on-demand, as needed by agents. This capability offers several benefits:
- Efficiency: Only the necessary tools are loaded into memory, optimizing resource utilization.
- Flexibility: New tools can be added to the system without requiring a restart or redeployment.
- Adaptability: Agents can dynamically adjust their capabilities based on the evolving demands of their tasks.
XMPro MAGS demonstrates dynamic tool loading, allowing for easy expansion of agent capabilities without modifying core code. This ensures that the system remains adaptable and can readily incorporate new tools as they become available or as the needs of the agents change.
Tool Usage Tracking
Tool usage tracking involves monitoring and recording the usage patterns and performance of different tools within the MAGS platform. This tracking mechanism provides valuable insights into:
- Tool Effectiveness: Identify which tools are most frequently used and how effectively they contribute to agent performance.
- Optimization Opportunities: Pinpoint areas where tool usage can be optimized, such as selecting more efficient tools or refining tool parameters.
- Cost Management: Track the costs associated with using external tools or APIs, enabling better resource allocation and cost control.
XMPro MAGS incorporates tool usage tracking, allowing for the analysis and optimization of tool selection and usage over time. This ensures that agents are utilizing the most effective tools for their tasks and that the overall system operates efficiently.
Multi-tool support, with its Tool Library, dynamic tool loading, and usage tracking capabilities, is a key aspect of MAGS platforms. It empowers agents with the flexibility and adaptability needed to tackle complex, real-world problems, enhancing their decision-making and problem-solving capabilities. XMPro MAGS exemplifies these principles, offering a robust and extensible multi-tool ecosystem that contributes to its overall effectiveness in industrial and enterprise environments.
Communication and Integration
In Multi-Agent Generative Systems MAGS, effective communication and integration are essential for coordinating the actions of multiple agents and enabling them to interact with various internal and external systems. This involves establishing robust communication channels and providing a unified interface for agents to access diverse resources and information. A key part of this is the message broker, which acts as a central hub for routing messages between agents and other systems.
Message Brokers
MAGS platforms often support a variety of message brokers to cater to different needs and environments. These brokers can provide different features and performance characteristics, allowing organizations to choose the best fit for their specific requirements. Some popular choices include the following:
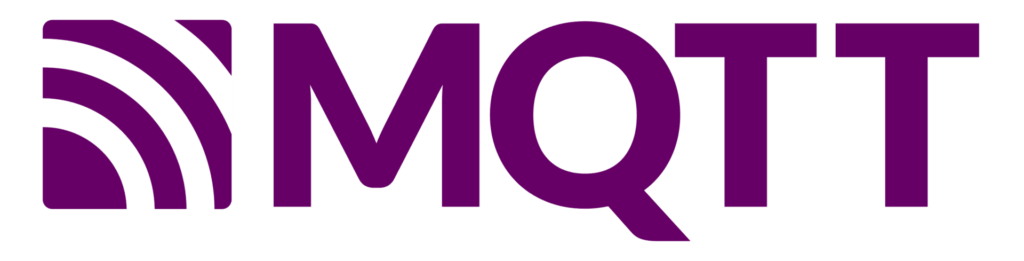
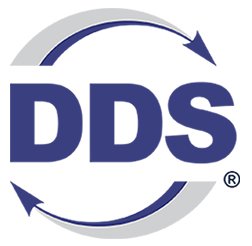
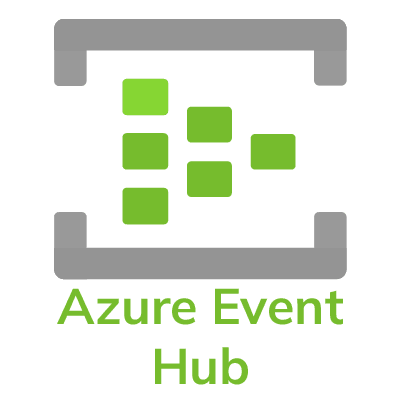
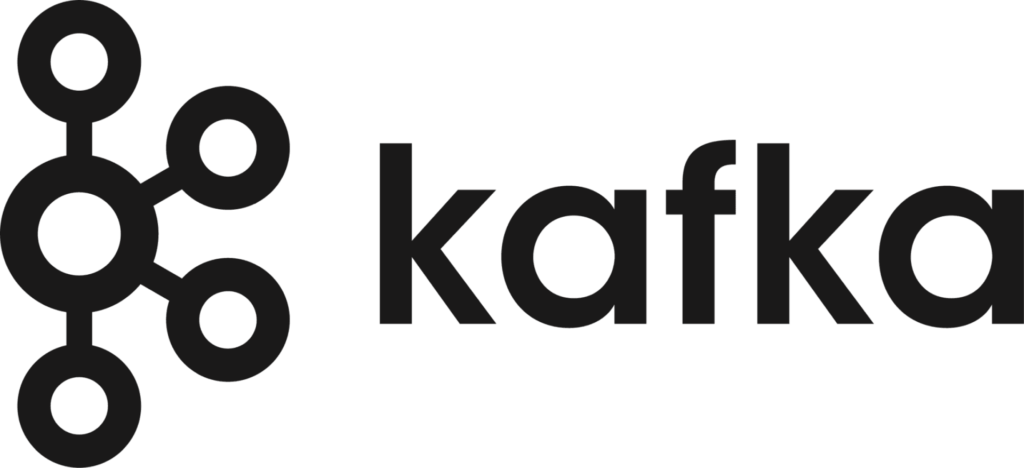
- MQTT (Message Queuing Telemetry Transport): A lightweight, publish-subscribe protocol widely used in IoT and industrial automation systems. It is suitable for resource-constrained devices and scenarios where efficient message delivery is crucial.
- DDS (Data Distribution Service): A real-time, data-centric protocol designed for high-performance and mission-critical applications. It offers features like deterministic data delivery and quality of service controls, making it suitable for demanding industrial environments.
- Kafka: A high-throughput, distributed streaming platform that can handle large volumes of data with low latency. It is well-suited for applications that require real-time data processing and analysis, such as log aggregation, stream processing, and event sourcing.
- Azure Event Hubs: A fully managed, real-time data ingestion service that is highly scalable and can handle millions of events per second. It is ideal for applications that require high throughput and low latency, such as telemetry data from devices, applications, and websites.
XMPro MAGS, for instance, supports MQTT, DDS, Kafka, and Azure Event Hubs protocols and can be integrated with various message brokers, providing flexibility in how agents communicate and integrate with various systems. This allows organizations to choose the most appropriate broker based on their specific needs, including factors like message volume, performance requirements, and existing infrastructure.
Abstracted Message Broker Interface
An abstracted message broker interface provides a unified way for agents to interact with different message brokers without needing to handle the complexities of each broker directly. This abstraction layer simplifies agent development and promotes interoperability between agents using different communication methods.
Key benefits of an abstracted message broker interface include the following:
- Simplified Agent Development: Agents can send and receive messages without needing to know the underlying communication protocol or the specific message broker being used.
- Flexibility: The system can easily switch or extend communication methods without affecting agent code, allowing for easy adaptation to new technologies or changing requirements.
- Interoperability: Agents using different protocols or connected to different brokers can seamlessly communicate with each other through the abstracted interface.
XMPro MAGS incorporates an abstracted message broker interface, allowing for easy switching or extension of communication methods. This ensures that agents can communicate effectively regardless of the underlying infrastructure and that the system remains adaptable to future communication technologies.
Support for multiple message brokers and the use of an abstracted message broker interface are key aspectsof MAGS platforms. They enable efficient and flexible communication between agents and various systems, promoting scalability, interoperability, and adaptability in complex operational environments. XMPro MAGS exemplifies these principles, providing a robust and flexible communication infrastructure that contributes to its overall effectiveness in industrial and enterprise settings.
III. Scaling MAGS in Complex Environments
Scaling Multi-Agent Generative Systems in complex environments requires a robust and adaptable architecture that can handle a growing number of agents, increasing data volumes, and evolving operational requirements. Key considerations for scalability include efficient agent management, flexible communication infrastructure, and the ability to handle complex decision-making processes.
Scalable Architecture
A scalable MAGS architecture should be designed to accommodate growth and change without compromising performance or efficiency. This involves:
Agent Profiles and Instances:
The separation of agent profiles (templates) and instances allows for easy scaling and management of multiple agents based on predefined templates. This architecture facilitates the deployment of numerous specialized agents across various roles and functions. XMPro MAGS, for example, employs this approach, enabling efficient management and deployment of agents based on predefined profiles.
Service-Oriented Architecture:
The use of dependency injection and service provider patterns throughout the codebase allows for modular design and easy extension of functionality. This approach promotes scalability by enabling the addition or removal of components without affecting the overall system architecture. XMPro MAGS utilizes a service-oriented architecture, allowing for flexible integration of new components and services as the system scales.
Decoupled Communication:
Agents should be able to communicate efficiently without creating dependencies or bottlenecks. Asynchronous communication patterns and message queues can help achieve decoupling and improve scalability. XMPro MAGS leverages MQTT and DDS protocols, which support decoupled communication and are designed for scalability in industrial environments.
Distributed Infrastructure:
For very large-scale deployments, consider a distributed infrastructure where agents and data are spread across multiple nodes or servers. This can improve performance and resilience by preventing overload on any single machine. XMPro’s stream host capabilities and support for edge computing enable distributed deployments and efficient data handling in large-scale industrial settings.
Advanced Planning and Decision-Making
In Multi-Agent Generative Systems, advanced planning and decision-making capabilities are crucial for enabling agents to effectively navigate complex scenarios and achieve their goals. This involves going beyond simple reactive behaviors and incorporating more sophisticated methods for strategizing, optimizing actions, and coordinating with other agents.
Multiple Planning Strategies
MAGS platforms often support multiple planning strategies to provide agents with the flexibility to handle diverse situations. These strategies may include the following:
- Plan-and-Solve: A common approach where agents generate a plan and then execute it, adapting to unexpected situations as they arise.
- Reactive Planning: Agents continuously monitor their environment and adjust their plans in real-time based on new observations.
- Goal-Directed Planning: Agents focus on achieving specific goals, breaking down complex goals into smaller, manageable sub-goals.
- Collaborative Planning: Agents coordinate their plans with other agents, sharing information and resources to achieve common objectives.
XMPro MAGS, for instance, incorporates a PlanningStrategyFactory and various strategy classes (like PlanAndSolveStrategy) to enable agents to employ different planning approaches based on the situation. This adaptability is crucial for handling the diverse challenges that agents may encounter in complex operational environments.
PDDL Integration
PDDL (Planning Domain Definition Language) is a standardized language for representing planning problems and domains. Integrating PDDL into MAGS platforms allows for:
- Standardized Representation: Define planning problems in a clear and consistent format that can be understood by different agents and systems.
- Complex Reasoning: Enable agents to reason about actions, preconditions, and effects in a structured manner.
- Interoperability: Facilitate the exchange of planning knowledge and models between different MAGS platforms or agents.
XMPro MAGS demonstrates PDDL integration in its Plan class, showcasing its support for standardized planning representations and complex reasoning about actions and goals. This capability enhances the agent’s ability to handle intricate planning tasks and collaborate with other agents using the same standardized language.
Incorporating multiple planning strategies and integrating PDDL into MAGS platforms are essential steps toward enabling advanced planning and decision-making capabilities in AI agents. These features enhance the agent’s ability to handle complex scenarios, adapt to changing conditions, and collaborate effectively with other agents. XMPro MAGS exemplifies these principles, providing a robust and flexible planning framework that contributes to its overall effectiveness in industrial and enterprise environments.
Handling Data at Scale
Multi-Agent Generative Systems, especially in complex environments, must handle large volumes of data efficiently. This requires a robust data management strategy that can accommodate diverse data types, complex relationships, and evolving information needs. Integrating graph databases and vector databases is crucial for achieving scalability and enabling agents to make informed decisions based on comprehensive data analysis.
Graph Database Integration
Graph databases excel at storing and querying complex, interconnected data structures, making them ideal for representing relationships between entities in a MAGS. Key benefits of integrating graph databases are:
- Complex Relationship Modeling:
- Effectively represent relationships between agents, memories, plans, and other entities in the MAGS.
- Capture the intricate dependencies and interactions within complex systems.
- XMPro MAGS utilizes Neo4j for graph-based data storage, enabling it to model the complex relationships inherent in industrial environments.
- Efficient Querying of Relationships:
- Traverse relationships and find patterns in connected data efficiently.
- Support complex queries that involve multiple levels of relationships, crucial for decision-making and planning in intricate scenarios.
- Maintaining Knowledge Structure:
- Store and manage the overall knowledge structure and meta-information about the agent’s experiences.
- Provide context and historical information for decision-making.
Vector Database Integration
Vector databases are specialized for storing and querying high-dimensional vector representations of data. Their key benefits in a MAGS are:
- Efficient Similarity-Based Retrieval:
- Enable rapid retrieval of information based on semantic similarity.
- Support efficient nearest-neighbor searches, crucial for finding relevant information in large datasets.
- XMPro MAGS incorporates multiple vector database options (Milvus, Qdrant, MongoDB Atlas) for this purpose.
- Context-Aware Information Retrieval:
- Facilitate context-aware information retrieval for language model prompts, enabling more effective use of generative AI capabilities.
- Supporting Advanced AI Operations:
- Store vector embeddings of memories, observations, and knowledge, enabling efficient implementation of retrieval-augmented generation (RAG) techniques.
Working Together for Comprehensive Data Management
Graph and vector databases work together to provide a comprehensive data management solution for MAGS:
- Semantic Retrieval and Structured Context: Vector databases enable the retrieval of semantically similar information, while graph databases provide the structured context and relationships surrounding that information.
- Hybrid Querying: Complex queries can leverage both semantic similarity and structured relationships, combining the strengths of both database types.
- Efficient Storage and Retrieval: Vector databases handle large volumes of unstructured data, while graph databases efficiently manage structured metadata and relationships.
By integrating both graph and vector databases, MAGS platforms like XMPro can effectively handle the data challenges of complex, large-scale environments. This approach enables agents to make more informed decisions based on a holistic understanding of the data, contributing to the overall intelligence and scalability of the system.
IV. Managing Observability in MAGS
Observability is crucial for managing and optimizing the performance of Multi-Agent Generative Systems, especially in complex, large-scale environments. It involves collecting, correlating, and analyzing data from various components of the system to gain insights into its behavior, identify potential issues, and ensure efficient operation.
OpenTelemetry Integration
OpenTelemetry is an open-source observability framework that provides a standardized way to collect and export telemetry data, including metrics, traces, and logs. Integrating OpenTelemetry into MAGS platforms allows for:
- Comprehensive Data Collection: Capture data from various components, including agents, message brokers, databases, and language models.
- Unified Data Format: Standardize telemetry data in a common format, facilitating analysis and correlation across different components.
- Extensibility: Easily integrate with various monitoring and analysis tools that support OpenTelemetry.
XMPro MAGS, for instance, demonstrates comprehensive integration with OpenTelemetry through its OpenTelemetrySetup class. This enables detailed performance tracking and analysis of the system’s behavior in a standardized and extensible manner.
Custom Metrics
In addition to standard telemetry data, MAGS platforms often benefit from collecting custom metrics specific to their operation and goals. These metrics can provide deeper insights into agent behavior, resource utilization, and overall system performance.
Examples of custom metrics in XMPro MAGS:
- LLMMetrics: Track the performance and usage of language models, including token consumption, response times, and error rates.
- ToolMetrics: Monitor the usage and effectiveness of different tools in the agent’s tool library, identifying optimization opportunities.
- Agent-Specific Metrics: Capture metrics related to individual agent performance, such as task completion rates, decision quality, and resource consumption.
Monitoring and Analysis Tools
Effective observability relies on the use of appropriate monitoring and analysis tools to visualize, analyze, and interpret the collected telemetry data. These tools can help identify trends, anomalies, and potential issues, enabling proactive management and optimization of the MAGS.
XMPro APEX, the AgentOps platform for XMPro MAGS, provides a suite of monitoring and analysis tools that allow users to:
- Visualize Agent Interactions: Observe the interactions between agents and various system components through dashboards and visualizations.
- Track Performance Metrics: Monitor key performance indicators (KPIs) related to agent behavior, resource utilization, and overall system health.
- Analyze Logs and Traces: Investigate issues and understand agent behavior by analyzing detailed logs and traces of agent actions and interactions.
- Set Alerts and Notifications: Configure alerts to be notified of critical events or anomalies, enabling proactive intervention and issue resolution.
By combining OpenTelemetry integration, custom metrics, and comprehensive monitoring tools, MAGS platforms like XMPro can achieve a high level of observability. This enables organizations to effectively manage and optimize their AI agent deployments, ensuring efficient operation, identifying potential issues, and maximizing the value of their MAGS investment.
V. Rules of Engagement and Ethical Considerations
Deontic Rules
In the context of Multi-Agent Generative Systems, deontic rules are used to define and enforce ethical boundaries for AI agent behavior. These rules specify what actions are permitted, obligatory, or forbidden for agents, ensuring that they operate responsibly and align with organizational values and societal norms.
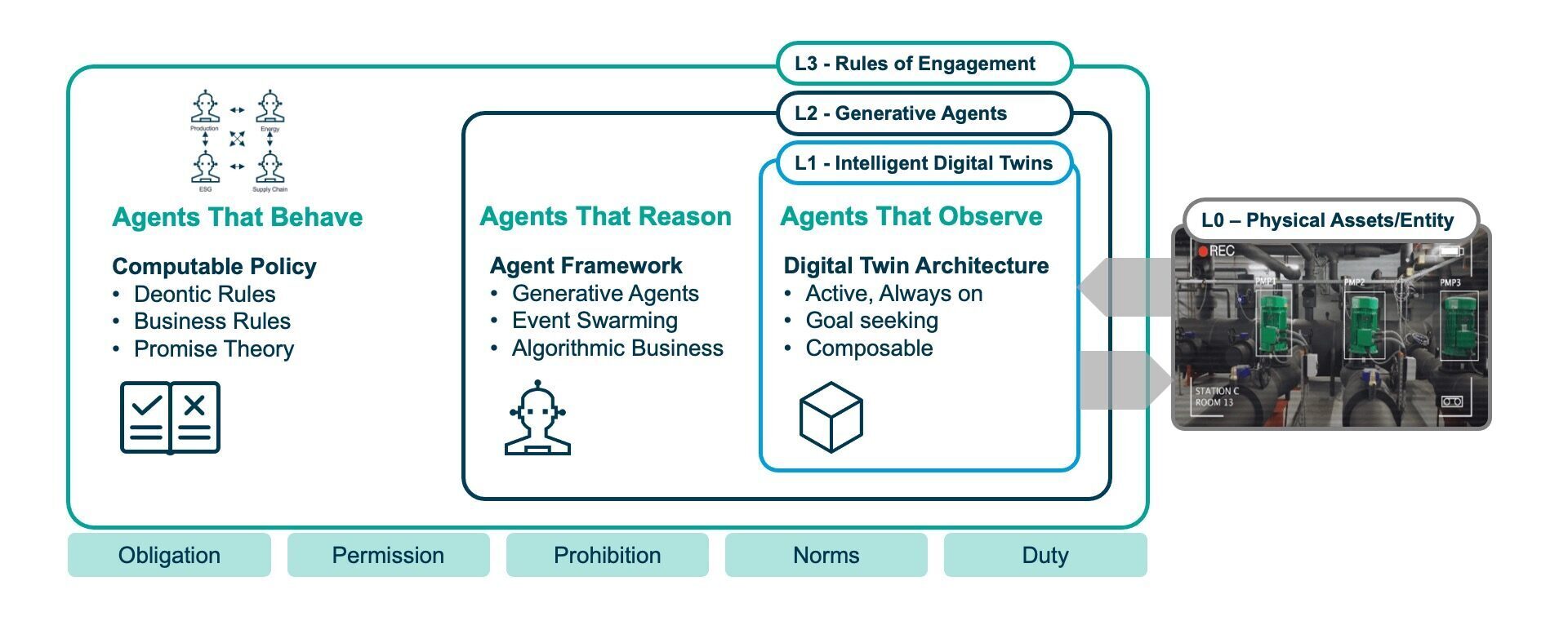
Defining Permitted, Obligatory, and Forbidden Actions
Deontic logic provides a framework for expressing different types of deontic rules:
- Permitted Actions (P): Actions that are allowed but not required for the agent.
- Obligatory Actions (O): Actions that the agent must perform under specific conditions.
- Forbidden Actions (F): Actions that the agent is strictly prohibited from performing.
These rules can be combined to create complex deontic constraints, ensuring that agents act ethically and in accordance with predefined guidelines.
Implementing Deontic Rules in Agent Profiles
Deontic rules can be implemented in MAGS agent profiles, providing a mechanism for defining and enforcing ethical behavior at the agent level. This involves:
- Formalizing Deontic Rules: Expressing deontic rules in a formal language that can be understood and interpreted by the MAGS platform.
- Integrating Rules into Agent Profiles: Incorporating deontic rules into the agent’s profile, specifying the permitted, obligatory, and forbidden actions for that particular agent.
- Enforcing Deontic Constraints: Implementing mechanisms within the MAGS platform to monitor agent actions and enforce the defined deontic rules.
XMPro MAGS, for instance, incorporates deontic rules into its agent profiles, ensuring that agents operate within ethical and organizational boundaries. This feature allows organizations to define and enforce responsible AI practices, promoting trust and transparency in their AI agent deployments.
Benefits of Implementing Deontic Rules
- Ethical AI: Ensure that AI agents act responsibly and ethically, minimizing the risk of unintended consequences or harm.
- Compliance: Enforce compliance with organizational policies, industry regulations, and societal norms.
- Trust and Transparency: Promote trust in AI systems by providing clear guidelines for agent behavior and demonstrating a commitment to ethical AI practices.
- Accountability: Establish accountability for AI agent actions, enabling organizations to track and audit agent behavior for compliance and ethical considerations.
In conclusion, incorporating deontic rules into MAGS agent profiles is a key step toward building responsible and ethical AI systems. It provides a mechanism for defining and enforcing clear boundaries for agent behavior, ensuring that AI agents operate in a manner that aligns with organizational values and societal expectations. XMPro MAGS exemplifies this principle, demonstrating its commitment to ethical AI practices and providing organizations with the tools to deploy AI agents responsibly.
Organizational Policies
Aligning Agent Behavior with Corporate Governance
In the context of Multi-Agent Generative Systems, organizational policies play a crucial role in ensuring that AI agents operate in alignment with corporate governance principles and internal guidelines. These policies provide a framework for responsible AI development and deployment, addressing ethical considerations, risk management, and compliance requirements.
Key aspects of aligning agent behavior with corporate governance:
- Policy Integration: Incorporating organizational policies into the MAGS framework, ensuring that agents are aware of and adhere to internal guidelines and standards.
- Access Control: Implementing access control mechanisms to restrict agent access to sensitive data and resources, preventing unauthorized actions or data breaches.
- Transparency and Explainability: Promoting transparency in agent decision-making processes and providing explanations for agent actions to foster trust and accountability.
- Risk Management: Identifying and mitigating potential risks associated with AI agent deployment, such as bias, discrimination, or unintended consequences.
- Human Oversight: Establishing mechanisms for human oversight and intervention in agent actions, especially in critical or high-risk scenarios.
Integrating Industry Regulations and Compliance Standards
In addition to internal policies, MAGS must also comply with relevant industry regulations and compliance standards. This involves:
- Regulatory Awareness: Staying informed about applicable regulations and standards, such as data privacy laws, industry-specific guidelines, and ethical AI frameworks.
- Compliance Implementation: Incorporating compliance measures into the MAGS design and operation, ensuring that agents adhere to legal and regulatory requirements.
- Auditing and Reporting: Implementing mechanisms for auditing agent behavior and generating reports to demonstrate compliance with relevant regulations and standards.
XMPro MAGS, with its emphasis on governance and ethical AI, provides features to support integrating organizational policies and industry regulations. This allows organizations to deploy AI agents responsibly, ensuring compliance and minimizing potential risks.
Benefits of Integrating Organizational Policies and Industry Regulations
- Responsible AI: Promote the responsible development and use of AI, minimizing potential harm and ensuring ethical considerations are addressed.
- Risk Mitigation: Reduce the risk of legal, financial, or reputational damage by ensuring compliance with relevant regulations and standards.
- Trust and Confidence: Build trust and confidence in AI systems by demonstrating a commitment to ethical AI practices and responsible governance.
- Operational Efficiency: Streamline compliance processes and reduce the burden of manual oversight by integrating policies and regulations into the MAGS framework.
In conclusion, aligning agent behavior with corporate governance and integrating industry regulations are essential steps toward building trustworthy and responsible MAGS. XMPro MAGS, with its focus on governance and ethical AI, provides organizations with the tools and capabilities to achieve these goals, ensuring that their AI agent deployments are compliant, ethical, and aligned with their business objectives.
Controlled Tool Access
In Multi-Agent Generative Systems, controlled tool access is a critical aspect of ensuring responsible and secure AI operations. It involves regulating which agents have permission to utilize specific tools within the system, preventing unauthorized or potentially harmful actions.
Key Mechanisms for Controlled Tool Access
- Role-Based Access Control (RBAC): Assign agents to specific roles with predefined permissions, determining which tools they can access based on their designated responsibilities.
- Attribute-Based Access Control (ABAC): Define fine-grained access control policies based on attributes of the agent, the tool, and the current context. This allows for more nuanced and dynamic control over tool access.
- Tool Permissions: Implement a permission system for individual tools, specifying which agents or roles are authorized to use them.
- Centralized Tool Management: Utilize a centralized tool library or registry to manage and control access to all tools within the MAGS platform.
- Auditing and Logging: Maintain detailed logs of tool usage, including which agents accessed specific tools and when, for monitoring and accountability purposes.
XMPro MAGS and Controlled Tool Access
XMPro MAGS incorporates controlled tool access through its ToolLibrary class, which manages tool permissions based on agent profiles. This ensures that agents only use tools they are authorized to access, preventing unauthorized actions and promoting responsible AI usage.
Benefits of Controlled Tool Access
- Security: Prevent unauthorized access to sensitive tools or resources, minimizing the risk of malicious activities or accidental harm.
- Risk Mitigation: Reduce the potential for unintended consequences or misuse of powerful AI tools by restricting access to authorized agents.
- Compliance: Enforce compliance with organizational policies, industry regulations, and ethical guidelines regarding AI tool usage.
- Operational Efficiency: Optimize tool utilization by ensuring that agents only have access to the tools necessary for their specific tasks.
- Accountability: Establish clear accountability for tool usage, enabling organizations to track and audit agent actions for compliance and ethical considerations.
Implementing Controlled Tool Access
- Identify Critical Tools: Determine which tools require controlled access based on their potential impact and sensitivity.
- Define Access Policies: Establish clear access control policies based on agent roles, attributes, or other relevant criteria.
- Implement Access Control Mechanisms: Integrate access control mechanisms into the MAGS platform, ensuring that agents cannot bypass restrictions.
- Monitor and Audit Tool Usage: Regularly monitor tool usage logs to identify potential violations or areas for improvement in access control policies.
In conclusion, controlled tool access is a fundamental aspect of responsible AI development and deployment in MAGS. By implementing appropriate access control mechanisms, organizations can ensure that AI agents operate safely, ethically, and in compliance with relevant guidelines. XMPro MAGS, with its focus on governance and ethical AI, provides the tools and capabilities to achieve controlled tool access, promoting trust and transparency in AI agent deployments.
VI. AgentOps Platform Services
XMPro APEX
XMPro APEX (Agent Platform EXperience) is a comprehensive Agent Operations (AgentOps) platform specifically designed to manage and optimize the performance of AI agents in Multi-Agent Generative Systems MAGS, especially within industrial and enterprise environments. It provides a suite of tools and services that streamline the development, deployment, monitoring, and governance of AI agents, enabling organizations to effectively leverage AI at scale.
Key services offered by XMPro APEX:
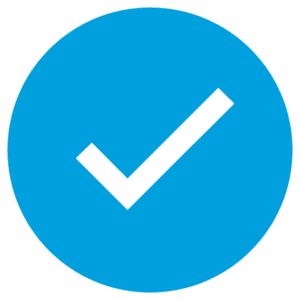
Agent Lifecycle Management
- Oversees the entire lifecycle of AI agents, from development and deployment to monitoring and optimization.
- Provides tools for creating, testing, deploying, and managing agents across different environments.
- Facilitates agent development, version control, and collaboration among developers.
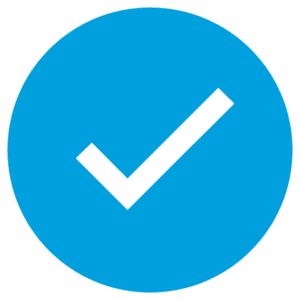
Interaction Analysis
- Analyzes interactions between different AI agents in the MAGS, helping developers understand how agents collaborate and identifying areas for improving inter-agent communication and cooperation.
- Facilitates the identification of communication patterns, potential conflicts, and opportunities for enhancing agent coordination.
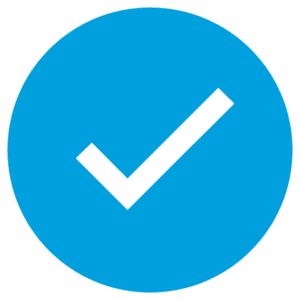
Cost Management
- Tracks and manages the costs associated with running MAGS, including LLM usage and computational resources.
- Provides detailed breakdowns of expenses and offers suggestions for optimizing cost-effectiveness.
- Enables organizations to monitor and control AI operational costs, ensuring efficient resource allocation.
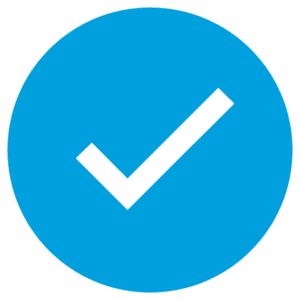
Performance Monitoring
- Offers real-time monitoring of agent performance, tracking metrics such as response times, success rates, and resource utilization.
- Enables quick identification of performance bottlenecks and areas for optimization.
- Provides insights into agent behavior and efficiency, allowing for data-driven improvements.
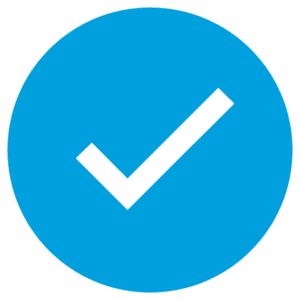
Error Detection & Debugging
- Includes advanced error detection capabilities, such as identifying infinite loops in agent reasoning or token limit overflows.
- Provides detailed logs and real-time alerting for common agent failures, facilitating efficient debugging and issue resolution.
- Helps developers identify and address errors quickly, minimizing downtime and ensuring smooth operation.
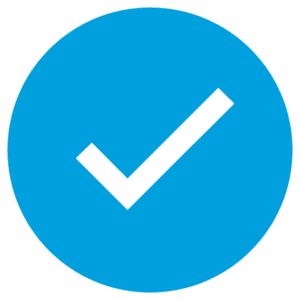
Governance & Compliance
- Incorporates a governance framework with deontic rules and organizational policies, ensuring that AI agents operate within defined boundaries and comply with corporate and industry regulations.
- Provides tools for enforcing ethical guidelines, managing risk, and ensuring responsible AI usage.
- Helps organizations maintain compliance with relevant regulations and standards, minimizing potential legal or ethical issues.
XMPro APEX’s comprehensive suite of AgentOps services empowers organizations to effectively manage and scale their AI agent deployments, ensuring optimal performance, responsible AI usage, and alignment with business objectives.
Other AgentOps Platforms
While XMPro APEX is a comprehensive and robust AgentOps platform, it’s not the only solution available. Several other platforms cater to the development, deployment, and management of AI agents, each with its strengths and focus areas.
Overview of Alternative Platforms
Here are some notable AgentOps platforms:
- Cogment: An open-source platform designed for building and deploying collaborative AI agents, particularly in real-time and interactive environments.
- AnyScale: A platform focused on the scalability and deployment of AI agents across various cloud and edge environments.
- Agents.dev: A platform that simplifies the development and deployment of AI agents by providing pre-built components and integrations.
- Arthur: An AI performance monitoring and observability platform that helps track and improve the performance of AI models and agents.
- Arize Phoenix: A platform specifically designed for monitoring and troubleshooting large language models (LLMs), offering tools for observability and explainability.
Choosing the Right AgentOps Platform
Selecting the most suitable AgentOps platform depends on several factors, includingu
- Specific needs and requirements: Consider the specific features and capabilities that are most important for your use case.
- Scalability needs: Evaluate the platform’s ability to handle the expected number of agents and data volume.
- Integration with existing systems: Ensure that the platform can seamlessly integrate with your current infrastructure and tools.
- Cost: Compare the pricing and cost-effectiveness of different platforms.
- Ease of use: Choose a platform that aligns with your team’s technical expertise and development preferences.
XMPro APEX stands out with its comprehensive suite of features, industrial focus, and strong emphasis on governance and ethical AI. However, other platforms may be more suitable for specific use cases or organizational preferences. Carefully evaluate your needs and consider the strengths of each platform before making a decision.
VII. XMPro MAGS: A Unique Approach
XMPro MAGS distinguishes itself from other platforms through its advanced cognitive capabilities, robust architecture, and comprehensive AgentOps features. It offers a unique approach to generative AI and multi-agent systems, specifically designed for the complexities of industrial and enterprise environments.
Cognitive Decision-Making with the Memory Cycle
One of the key differentiators of XMPro MAGS is its sophisticated approach to cognitive decision-making, powered by a unique Memory Cycle. This cycle mimics human-like reasoning processes, guiding agents through four key stages: observe, reflect, plan, and act.
- Observe: Agents continuously gather information from their environment, including real-time data streams, sensor readings, and interactions with other agents. This observational data is processed and stored in the agent’s memory, forming the basis for future decision-making.
- Reflect: Agents analyze their accumulated observations, identifying patterns, trends, and anomalies. They leverage historical data and contextual information to gain a deeper understanding of the situation and evaluate potential outcomes.
- Plan: Based on their reflections, agents formulate plans of action to achieve their goals. These plans may involve coordinating with other agents, accessing external tools, or generating creative solutions using generative AI capabilities.
- Act: Agents execute their plans, interacting with their environment and taking actions based on their decisions. The outcomes of these actions are then observed and fed back into the Memory Cycle, allowing for continuous learning and adaptation.
This cyclical process enables continuous learning and dynamic adaptation, allowing agents to adjust their strategies and improve their decision-making over time. It also fosters contextual awareness, as agents consider historical data and current observations when making decisions.
Key Benefits of the Memory Cycle:
- Contextual Awareness: Agents make decisions that are contextually relevant and informed by past experiences.
- Continuous Learning: Agents learn from their actions, adapting their strategies and improving their decision-making over time.
- Dynamic Adaptation: Agents respond effectively to changing conditions and unexpected events.
- Cognitive Depth: Agents perform complex reasoning, considering multiple factors and potential outcomes before making decisions.
Why XMPro MAGS Stands Out
Here’s how XMPro MAGS compares to other agent frameworks like LangGraph, AutoGen, and CrewAI, highlighting its unique strengths in addressing the complexities of industrial environments:
- Advanced Cognitive Architecture: Unlike frameworks that rely on predefined workflows and rules, XMPro MAGS employs a cognitive architecture that enables agents to learn and adapt in dynamic situations.
- Industrial-Grade Messaging: XMPro MAGS utilizes robust messaging protocols like MQTT and DDS, ensuring reliable communication in demanding industrial settings where other frameworks might rely on less robust HTTP-based messaging.
- Dual-Database Systems: XMPro MAGS combines vector and graph databases for comprehensive data handling, enabling both semantic searches and an understanding of complex relationships between data points. This is crucial for informed decision-making in data-intensive industrial environments.
- Continuous Learning: XMPro MAGS agents learn from their actions and adapt their behavior over time, reducing the need for manual intervention and increasing resilience to changing conditions. This contrasts with static workflows that require frequent updates.
- Comprehensive Management with APEX: XMPro’s APEX platform provides comprehensive lifecycle management for AI agents, bridging the gap between OT and IT systems for seamless integration of AI-driven decisions.
Deep Integration with Industrial Systems
XMPro MAGS is purpose-built for industrial challenges. Its deep integration with industrial systems is a key differentiator, enabling seamless connectivity with operational technology (OT) and information technology (IT) infrastructure.
Data Streaming
XMPro MAGS excels at handling real-time data streams from various industrial sources, including sensors, machines, and control systems. This capability is crucial for enabling agents to:
- Monitor real-time conditions and respond quickly to events.
- Analyze operational data to identify patterns and trends.
- Make informed decisions based on the latest information.
The platform’s support for MQTT and DDS messaging protocols ensures reliable and efficient data streaming in industrial settings.
Digital Twin Potential
XMPro MAGS’ comprehensive memory and planning systems, coupled with its data streaming capabilities, provide a strong foundation for creating and integrating with digital twins. Digital twins are virtual representations of physical assets or processes, enabling:
- Simulation and Modeling: Test different scenarios and predict outcomes in a risk-free environment.
- Predictive Maintenance: Anticipate equipment failures and optimize maintenance schedules.
- Process Optimization: Identify bottlenecks and inefficiencies in industrial processes.
- Real-Time Monitoring: Track the performance and health of physical assets in real time.
XMPro’s expertise in creating digital twins, combined with the capabilities of MAGS, allows organizations to build sophisticated digital representations of their operations and leverage AI agents for advanced analysis, prediction, and optimization.
Key Benefits of Deep Industrial Integration:
- Enhanced Operational Efficiency: Real-time data analysis and AI-driven insights lead to improved operational efficiency and productivity.
- Predictive Maintenance: Reduce downtime and maintenance costs by anticipating equipment failures and optimizing maintenance schedules.
- Process Optimization: Identify and address bottlenecks and inefficiencies in industrial processes, leading to improved throughput and resource utilization.
- Innovation and Agility: Digital twin simulations enable rapid innovation and agile responses to changing market demands.
- Data-Driven Decision-Making: Real-time data and AI-powered insights support informed decision-making across all levels of the organization.
XMPro MAGS’ deep integration with industrial systems empowers organizations to transform their operations, leveraging the power of AI and digital twins to achieve operational excellence and drive innovation.
Natural Language Processing Integration
Multi-Agent Generative Systems MAGS often leverage natural language processing (NLP) to enable agents to understand, interpret, and generate human-like text. This capability is crucial for tasks such as:
- Human-Agent Communication: Allow agents to interact with humans using natural language, facilitating more intuitive and efficient communication.
- Textual Data Analysis: Analyze and extract insights from textual data sources, such as documents, reports, and web pages.
- Content Generation: Generate human-quality text for various purposes, including reports, summaries, and creative content.
Model Agnostic Design
XMPro MAGS adopts a model-agnostic design for its NLP integration, meaning that the system can work with different language models and providers, including OpenAI, Azure, Anthropic, and Google. This approach offers several benefits:
- Flexibility: Adapt to the evolving landscape of language models, allowing organizations to choose the best model for their specific needs and preferences.
- Future-Proofing: Avoid vendor lock-in and ensure compatibility with future advancements in NLP technology.
- Performance Optimization: Select the most suitable language model for different tasks based on performance, cost, or other criteria.
Sophisticated Prompt Management
XMPro MAGS incorporates a robust prompt management system that enables dynamic management and optimization of prompts for different tasks. This system allows for:
- Prompt Engineering: Design and refine prompts to elicit the desired responses from language models.
- Contextualization: Incorporate relevant context and information into prompts to improve the accuracy and relevance of generated text.
- Prompt Versioning: Track and manage different versions of prompts for experimentation and optimization.
Key Benefits of NLP Integration:
- Enhanced Communication: Facilitate more natural and efficient communication between agents and humans.
- Improved Decision-Making: Leverage insights from textual data analysis to support informed decision-making.
- Automated Content Creation: Generate high-quality text for various purposes, improving productivity and efficiency.
- Adaptability and Flexibility: Utilize different language models and optimize prompts for specific tasks, ensuring the system remains adaptable and effective.
XMPro MAGS’ NLP integration, with its model-agnostic design and sophisticated prompt management, showcases the platform’s commitment to providing comprehensive and flexible AI capabilities for complex industrial and enterprise environments.
Extensibility and Customization
In the dynamic landscape of AI and industrial automation, extensibility and customization are crucial for Multi-Agent Generative Systems MAGS to adapt to evolving needs and specific industry requirements. XMPro MAGS recognizes this need and provides a flexible architecture that allows for easy extension and customization.
Abstract Base Classes
XMPro MAGS employs abstract base classes for many of its core components. This design approach allows developers to extend the functionality of the system by creating new classes that inherit from these abstract base classes. This enables the integration of new technologies, algorithms, or strategies without modifying the core codebase, promoting modularity and maintainability.
For example, the AbstractVector
and AbstractEmbedding
classes in XMPro MAGS provide a blueprint for implementing different vector representations and embedding methods. Developers can extend these classes to incorporate new techniques or adapt to specific data formats, ensuring the system remains flexible and adaptable to future advancements in AI.
Plugin Architecture
XMPro MAGS also utilizes a plugin-like architecture, particularly evident in its tool system. This allows developers to add new capabilities and tools to the system without altering the core code. New tools can be easily integrated as plugins, expanding the agent’s capabilities and allowing them to interact with different systems or perform specialized tasks.
This plugin approach promotes extensibility and customization by:
- Enabling the addition of new tools or functionalities without disrupting the core system.
- Allowing developers to create custom tools tailored to specific industry needs or unique operational requirements.
- Fostering a modular and maintainable codebase, where different components can be developed and updated independently.
Benefits of Extensibility and Customization
- Adaptability: Easily adapt to new technologies, algorithms, and industry-specific requirements.
- Flexibility: Customize the system to meet unique operational needs and integrate with various tools and platforms.
- Scalability: Extend the system’s capabilities as needed to handle growing data volumes and complex tasks.
- Innovation: Facilitate the development and integration of new AI techniques and tools, promoting innovation and continuous improvement.
XMPro MAGS’ extensible and customizable architecture ensures that the platform remains adaptable and relevant in the ever-evolving landscape of AI and industrial automation. This approach empowers organizations to tailor the system to their specific needs, integrate new technologies, and continuously optimize their AI agent deployments for maximum effectiveness.
VIII. Getting Started with MAGS
Building a MAGS system can seem daunting, but breaking down the process into key steps can make it more manageable. Here’s a guide to get you started:
Building a MAGS System
Defining Agent Roles and Interactions
- Identify the Problem: Clearly define the problem you want your MAGS to solve. This will guide your agent design and interactions.
- Define Agent Roles: Determine the different roles and responsibilities needed within your MAGS. Each agent should have a specific area of expertise or task to perform.
- Interaction Design: Map out how agents will interact and collaborate to solve the problem. Consider communication patterns, information sharing protocols, and decision-making hierarchies.
Designing the Communication Infrastructure
- Protocol Selection: Choose appropriate communication protocols based on your needs and environment. Consider factors such as real-time requirements, scalability, and security.
- Message Broker: Select a message broker that supports your chosen protocols and can handle the expected message volume and agent interactions.
- Communication Channels: Establish clear communication channels between agents, ensuring efficient and reliable message delivery.
Implementing the Memory Cycle
- Memory Representation: Determine how agent memories will be represented and stored. Consider using a combination of vector and graph databases to handle diverse data types and relationships.
- Memory Cycle Implementation: Implement the observe-reflect-plan-act loop for each agent, ensuring that they can learn from past experiences and adapt their behavior.
- Memory Sharing: If necessary, establish mechanisms for agents to share memories or access a shared knowledge base, facilitating collaboration and collective learning.
Additional Considerations
- Agent Capabilities: Determine the specific capabilities each agent needs, such as natural language processing, image recognition, or specialized tools.
- Scalability: Design the system to handle potential growth in the number of agents and data volume.
- Monitoring and Observability: Incorporate tools and techniques for monitoring agent behavior, system performance, and overall health.
- Governance and Ethics: Establish clear rules of engagement and ethical guidelines for agent behavior to ensure responsible AI usage.
Getting Started with XMPro
XMPro offers a comprehensive platform for building and deploying MAGS, including:
- XMPro DataStream: Ingest and process real-time data from various sources.
- XMPro AI: Build and train AI models for agent decision-making.
- XMPro APEX: Manage the lifecycle, integration, and optimization of MAGS agents.
XMPro’s platform provides a robust and user-friendly environment for building and deploying MAGS, enabling organizations to leverage the power of AI agents for complex problem-solving and operational efficiency.
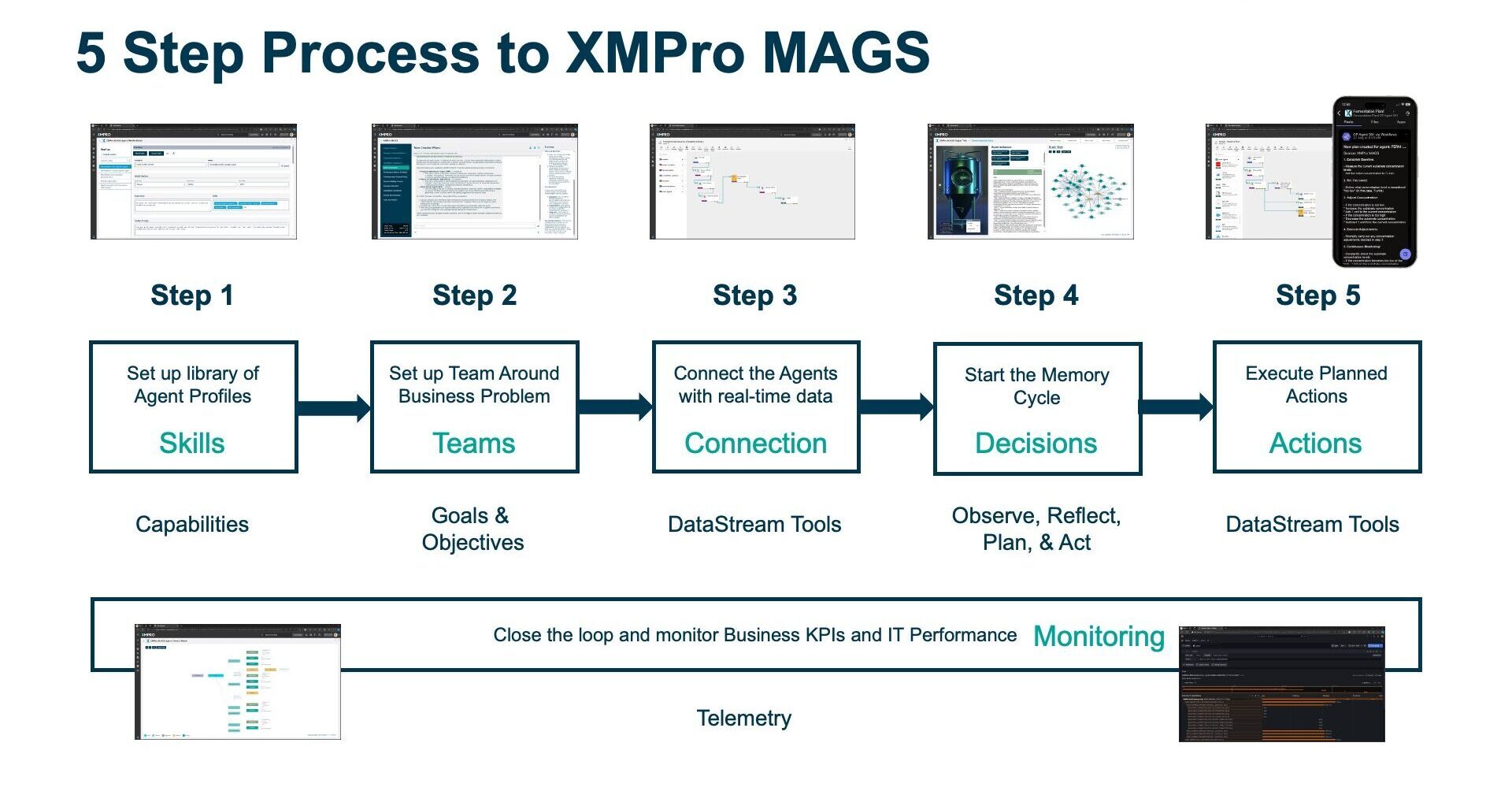
Deploying MAGS
Choosing an AgentOps Platform
An AgentOps platform provides the tools and infrastructure for managing and monitoring your MAGS deployment. Evaluate different platforms based on their features, scalability, ease of use, and integration capabilities. XMPro APEX is a comprehensive AgentOps platform specifically designed for industrial and enterprise environments, offering a suite of tools for agent lifecycle management, performance monitoring, governance, and more.
Integrating with Existing Systems
Seamless integration with your existing systems and data sources is crucial for successful MAGS deployment. Consider the following:
- Data Integration: Ensure that your MAGS can access and process data from various sources, including sensors, databases, and cloud platforms. XMPro DataStream facilitates data integration from diverse sources.
- Legacy System Integration: If necessary, bridge the gap between your MAGS and legacy systems using appropriate connectors or APIs.
- Workflow Integration: Incorporate your MAGS into existing operational workflows, ensuring that agents can interact with other systems and processes.
Monitoring and Optimizing Performance
Continuous monitoring and optimization are essential for ensuring the ongoing success of your MAGS deployment.
- Performance Monitoring: Track key performance indicators (KPIs) related to agent behavior, resource utilization, and overall system health. XMPro APEX provides tools for real-time performance monitoring and analysis.
- Error Detection and Debugging: Implement mechanisms for detecting and resolving errors or unexpected behavior in your MAGS. XMPro APEX offers advanced error detection and debugging capabilities.
- Optimization: Continuously analyze performance data and identify areas for optimization, such as refining agent decision-making, improving communication efficiency, or scaling resources.
Additional Deployment Considerations
- Security: Implement appropriate security measures to protect your MAGS from unauthorized access and cyber threats.
- Scalability: Ensure that your deployment can scale to handle future growth in data volume and agent interactions.
- Governance: Establish clear governance policies and procedures for managing your MAGS, ensuring responsible AI usage and compliance with regulations.
By carefully considering these deployment aspects, you can ensure that your MAGS system integrates smoothly into your operational environment and delivers the desired outcomes.
Use Cases and Examples
Multi-Agent Generative Systems MAGS have the potential to revolutionize various industries and applications by enabling the automation of complex tasks, creative problem-solving, and improved decision-making. Here are a few examples of how MAGS can be applied in different domains:
Industrial Process Control and Optimization
MAGS can be used to create intelligent and autonomous systems for industrial process control and optimization, leading to increased efficiency, productivity, and safety.
Use Cases and Examples
Multi-Agent Generative Systems MAGS have the potential to revolutionize various industries and applications by enabling the automation of complex tasks, creative problem-solving, and improved decision-making. Here are a few examples of how MAGS can be applied in different domains:
Industrial Process Control and Optimization
MAGS can be used to create intelligent and autonomous systems for industrial process control and optimization, leading to increased efficiency, productivity, and safety.
Examples:
- Process Parameter Optimization: AI agents can analyze historical data and real-time sensor readings to generate optimized process parameters, such as temperature, pressure, and flow rates, for various manufacturing processes.
- Advanced Process Control: Agents can develop and implement advanced control strategies, including complex control algorithms and dynamic parameter adjustments, to maintain optimal process conditions.
- Predictive Maintenance: AI agents can analyze equipment sensor data and maintenance logs to generate predictive maintenance schedules, reducing downtime and optimizing maintenance costs.
- Anomaly Detection and Root Cause Analysis: Agents can learn normal process behavior from historical data and detect anomalies in real-time sensor readings, providing hypotheses on potential root causes based on process knowledge.
- Simulation and Scenario Analysis: AI agents can simulate various process scenarios by generating synthetic data representing different operating conditions, equipment states, or process disturbances, enabling analysis and optimization before implementing changes.
- Knowledge Synthesis and Decision Support: Agents can ingest process documentation, manuals, and expert knowledge to synthesize information, provide decision support, generate troubleshooting guides, and create training materials for operators.
Tech Stack Monitoring and Management
MAGS can optimize and manage an organization’s technology infrastructure by monitoring performance, predicting maintenance needs, and recommending upgrades.
Examples:
Infrastructure Performance Optimization: AI agents can analyze and optimize the performance of various components in the tech stack, generating optimized configurations and providing real-time adjustments to maintain peak performance.
Advanced Control Strategy: Agents can develop and implement advanced strategies for managing the tech stack, creating complex control algorithms for resource allocation and implementing dynamic adjustments to maintain optimal system conditions.
Predictive Maintenance and Upgrade: AI agents can analyze system logs and performance data to predict potential issues, generating maintenance schedules to minimize downtime and recommending timely upgrades to improve system efficiency and security.
Anomaly Detection and Root Cause Analysis: Agents can monitor system behavior in real-time, identifying unusual patterns or performance issues, conducting root cause analysis, and generating potential solutions.
Simulation and Scenario Analysis: AI agents can simulate various scenarios to test potential changes or upgrades, creating synthetic data representing different tech stack configurations and generating reports on potential outcomes and risks.
Knowledge Synthesis and Decision Support: Agents can synthesize information from various sources to support decision-making, aggregating and analyzing data from all other agents, generating comprehensive reports and recommendations, and creating documentation and training materials for the tech team.
Water Utilities Systems
MAGS can automate the creation, curation, validation, auditing, and certification of system specifications for water utilities, improving efficiency, accuracy, and compliance while reducing manual effort.
Examples:
- Artifact Scanning and Context Preparation: AI agents can scan artifacts folders, identify relevant files for each water utilities component, and combine them into a single context file for comprehensive information.
- Specification Generation and Refinement: Agents can use AI models to generate initial draft specifications, post-process the content, and merge tables to create comprehensive specification documents.
- Table Generation: AI agents can generate tables for ports, parameters, and other essential data based on CSV files provided as inputs.
- Format Conversion and Distribution: Agents can convert specifications into different formats (e.g., HTML, PDF) and distribute them to relevant stakeholders.
- Review Coordination and Status Tracking: AI agents can coordinate the human review process and track the status of each specification in real-time.
- Edit Implementation and Regeneration: Agents can assist in making necessary edits and regenerate the specification incorporating human feedback.
XMPro MAGS for Industrial and Enterprise Applications
XMPro MAGS is particularly well-suited for complex industrial and enterprise environments, offering a robust and scalable platform for deploying AI agents in mission-critical applications. Its focus on industrial integration, cognitive decision-making, and continuous learning makes it a powerful tool for organizations looking to leverage the full potential of MAGS.
IX. Conclusion
The Future of MAGS and Generative AI
Multi-Agent Generative Systems MAGS represent a new frontier in AI, poised to transform industries and applications by enabling the automation of complex tasks, creative problem-solving, and improved decision-making. As generative AI models continue to advance, we can expect MAGS to become even more sophisticated and capable, driving innovation and efficiency across various domains.
XMPro’s Vision for Industrial AI Excellence
XMPro is committed to pioneering the future of industrial AI, and MAGS is a key component of that vision. XMPro MAGS is specifically designed to address the unique challenges of complex industrial and enterprise environments, offering a robust and scalable platform for deploying AI agents in mission-critical applications. Its focus on industrial integration, cognitive decision-making, and continuous learning makes it a powerful tool for organizations looking to leverage the full potential of MAGS.
Call to Action: Get Started with XMPro MAGS
If you’re looking to explore the potential of MAGS for your organization, XMPro offers a comprehensive suite of tools and services to get you started:
- XMPro DataStream: Ingest and process real-time data from various sources.
- XMPro AI: Build and train AI models for agent decision-making.
- XMPro APEX: Manage the lifecycle, integration, and optimization of MAGS agents.
XMPro’s platform provides a robust and user-friendly environment for building, deploying, and managing MAGS, enabling organizations to leverage the power of AI agents for complex problem-solving and operational efficiency. Contact XMPro today to learn more and start your journey towards a more intelligent and automated future.
X. Appendices
Glossary of Terms
- Agent: An AI entity capable of autonomous reasoning, decision-making, and action-taking within a MAGS.
- AgentOps: The operational framework and practices for managing and scaling AI agents.
- Generative AI: AI models that can generate new content and ideas, such as text, images, or code.
- Graph Database: A database that stores and manages interconnected data and relationships.
- LLM (Large Language Model): AI models trained on vast amounts of text data, capable of generating human-quality text and performing various language-based tasks.
- MAGS (Multi-Agent Generative Systems): A system of multiple AI agents that interact and collaborate using generative AI capabilities.
- Memory Cycle: The cognitive process in XMPro MAGS that enables agents to observe, reflect, plan, and act.
- MQTT: A lightweight messaging protocol commonly used in IoT and industrial automation.
- OpenTelemetry: An open-source observability framework for collecting and exporting telemetry data.
- PDDL (Planning Domain Definition Language): A standardized language for representing planning problems and domains.
- Vector Database: A database that stores and retrieves high-dimensional vector representations of data.
- XMPro Multi-Agent Generative Systems MAGS: MAGS (Multi-Agent Generative Systems) are dynamic teams of virtual workers powered by advanced artificial intelligence. These self-organizing teams work independently and collaboratively to optimize operational outcomes and achieve specified goals. Key features of MAGS include the following:
- Independence and Agency: Each virtual worker functions autonomously, making decisions and taking actions independently within their defined scope.
- Planning and Reflection: They can plan ahead, reflect on past actions, and adjust strategies accordingly, enabling continuous improvement.
- Anticipatory and Goal-Seeking: They proactively identify and work towards operational objectives, always striving for optimal outcomes.
- Always-On Collaboration: They operate 24/7, constantly monitoring, communicating, and responding to changes in their environment, ensuring seamless coordination.
- Adaptive Reasoning and Decision-Making: They use advanced AI to analyze situations, solve problems, and make informed choices, adapting their execution techniques based on inputs and environmental considerations.
- Complex Workflow Execution: They can handle and optimize intricate operational processes with minimal human intervention, streamlining complex tasks.
- Rules of Engagement: Agents and teams operate under a structured set of deontic principles, including obligations, permissions, prohibitions, conditional rules, and normative guidelines. These rules govern their behavior, ensure ethical operations, maintain safety standards, and facilitate effective collaboration while providing a framework for autonomous decision-making within predefined boundaries.
List of AgentOps Platforms
- XMPro APEX
- Cogment
- AnyScale
- Agents.dev
- Arthur
- Arize Phoenix
Resources and Further Reading
- XMPro Website: https://xmpro.com/generative-ai/
- Blog Article on AgentOps: Difference Between MAGS APEX and AgentOps.md
- Research Article on XMPro MAGS: MAGS vs CrewAI etc.md
- White Paper on MAGS in Industrial Automation: How XMPro MAGS qualify as a system based on Meadows analysis.md
- Book on Generative AI: https://books.google.com/books/about/Generative_AI_for_Business.html?id=QFKnzwEACAAJ